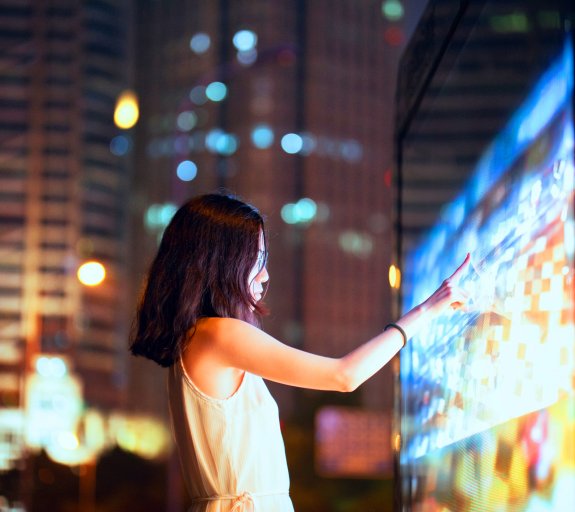
How understanding sentiment can help improve investment outcomes
- 28 April 2025 (7 min read)
KEY POINTS
Investor sentiment is an important factor when assessing the fundamental value of a stock. Analysing the sentiment of company management in an earnings call can give us valuable insights into the confidence and direction of corporate strategy.
On a fundamental level, positive messages from a company’s management team, regarding for example, upcoming product launches or key events, can potentially send its stock price higher; any negative messages can have the opposite effect.
Our sentiment model analysis the transcripts from company earnings calls and uses natural language processing (NLP) to parse the text in these documents, focusing on the important snippets of conversation to score how positive or negative company management is, and how significant the changes in language are since the previous call.
By actively monitoring sentiment in tandem with our core fundamental view of a stock, specifically around its quality and valuation, we can form a better view of the stock and its future fundamental potential. This holistic approach not only helps identify potential investment opportunities and risks but also supports more strategic decision-making in an ever-changing financial landscape.
Ultimately, understanding sentiment allows investors to align their strategies with market dynamics, enhancing their chances of achieving a favourable outcome.
What is a ‘sentiment’ model?
Sentiment as a factor encompasses various dimensions. This includes price sentiment - which reflects movements in stock prices - and earnings sentiment, which pertains to earnings revisions.
We look at NLP-based sentiment indicators, such as sentiment derived from earnings calls, news articles, and social media. NLP is a type of artificial intelligence (AI) that looks to decipher human language, and these models can classify text into categories such as positive, neutral, or negative sentiment. Some offer more granular classifications, distinguishing between sentiments like very negative, negative, neutral, positive, and very positive.
Early NLP models often relied on counting the frequency of positive, negative, and neutral words to classify text. The primary objective of NLP-based sentiment classification is to assess sentiment intensity. Recent advancements have led to the development of more sophisticated models that take context into account, which allow a deeper understanding of sentiment nuances.
How sentiment models have evolved
The Harvard IV-4 dictionary, one of the earliest tools for sentiment analysis, created in the 1990s, categorises words into general sentiment and psychological categories like ‘positive’ or ‘negative.’ Its broad design makes it versatile but limits its precision in terms of focusing on particular areas like finance.
The Loughran-McDonald model, meanwhile, was introduced in 2011 and relied on sentiment dictionaries that classify words according to their sentiment but was tailored to the financial sector. For instance, terms like ‘underperform’, ‘adverse’ and ‘unforeseen’ are labelled as negative, while words such as ‘profitable’, ‘outperform’ and ‘bullish’ are labelled as positive. Unlike general-purpose dictionaries, it captures nuanced financial meanings, defining terms like ‘liability’ or ‘risk’ as negative.
The model counts how often these words occur, and if positive words make up the highest proportion, the sentence is classified as positive.
Our first model used the Loughran-McDonald dictionary - its financial specialism has made it a key tool for analysing sentiment in financial texts and linking it to market behaviour and company performance.
More recently, transformer-based models like FinBERT have revolutionised sentiment analysis with deep learning and contextual embeddings. Transformer architecture was a major game-changer and driving force in the development of AI and was fundamental to the success of ChatGPT.
The key concept of the transformer is its self-attention mechanism, which allows the model to weigh the importance of different elements in the input sentence when making predictions. This attention mechanism enables the model to capture long-range dependencies and understand the context of each word in relation to the entire sentence. They have been able to capture complex patterns and deep relationships in language, reshaping the landscape of NLP.
FinBERT is specifically trained on financial data, allowing it to better understand the nuances of financial language, such as how ‘volatile’ implies risk. This model not only recognises sentiment but also comprehends the context in which words are used, capturing the deeper meanings associated with financial terms. As a result, FinBERT provides a significantly more accurate sentiment analysis in the financial domain compared to traditional dictionary-based models.
We employ a FinBERT sentiment model to analyse the transcribed text from companies’ quarterly earnings calls, using an NLP technique to determine if management are speaking with positive or negative sentiment. In our view, FinBERT has become a powerful tool for analysing complex financial texts, aiding market predictions and decision-making.
A comparison of sentiment model accuracy
In the charts below, we compare sentiment classification using the Loughran-McDonald dictionary, the Harvard IV-4 dictionary, and FinBERT.
We analysed 300 news articles from the Financial Times, manually classifying them as ‘positive,’ ‘negative,’ or ‘neutral.’ We then measured how well the three different approaches scored these documents. The results show very clear differences in the performance of the three models. FinBERT achieved the highest accuracy, correctly classifying 81.7% of titles, with only 18.3% incorrect. The Loughran-McDonald dictionary had 54.0% of classifications correct and 46.0% incorrect. The Harvard IV-4 dictionary had the lowest accuracy, with 45.0% correct classifications and 55.0% incorrect. In our view, these results underscore FinBERT's superior ability to accurately capture sentiment in financial texts compared to traditional dictionary-based methods.
Using sentiment analysis to potentially improve outcomes
As quantitative investors, we leverage NLP-based sentiment indicators as an important component in constructing our momentum factor. Currently, we incorporate NLP-based sentiment from earnings call transcripts into our proprietary momentum factor. Sentiment indicators can be combined with other factors to create a more comprehensive stock selection approach.
We believe that it is necessary to continuously enhance our models to maintain our stock selection advantage. We believe our experience in this field puts us in a good position to continue to take advantage of the latest developments in AI and machine learning to aim to deliver the best possible outcomes for our clients.
Subscribe to updates
Have our latest insights delivered straight to your inbox
Disclaimer
This document is for informational purposes only and does not constitute investment research or financial analysis relating to transactions in financial instruments as per MIF Directive (2014/65/EU), nor does it constitute on the part of AXA Investment Managers or its affiliated companies an offer to buy or sell any investments, products or services, and should not be considered as solicitation or investment, legal or tax advice, a recommendation for an investment strategy or a personalized recommendation to buy or sell securities.
Due to its simplification, this document is partial and opinions, estimates and forecasts herein are subjective and subject to change without notice. There is no guarantee forecasts made will come to pass. Data, figures, declarations, analysis, predictions and other information in this document is provided based on our state of knowledge at the time of creation of this document. Whilst every care is taken, no representation or warranty (including liability towards third parties), express or implied, is made as to the accuracy, reliability or completeness of the information contained herein. Reliance upon information in this material is at the sole discretion of the recipient. This material does not contain sufficient information to support an investment decision.
Issued in the UK by AXA Investment Managers UK Limited, which is authorised and regulated by the Financial Conduct Authority in the UK. Registered in England and Wales, No: 01431068. Registered Office: 22 Bishopsgate, London, EC2N 4BQ.
In other jurisdictions, this document is issued by AXA Investment Managers SA’s affiliates in those countries.
© 2025 AXA Investment Managers. All rights reserved
Image source: Getty Images
Risk Warning
The value of investments, and the income from them, can fall as well as rise and investors may not get back the amount originally invested.